AI Bias: The Hidden Dangers in Machine Learning Algorithms - Unveiling biases in AI systems
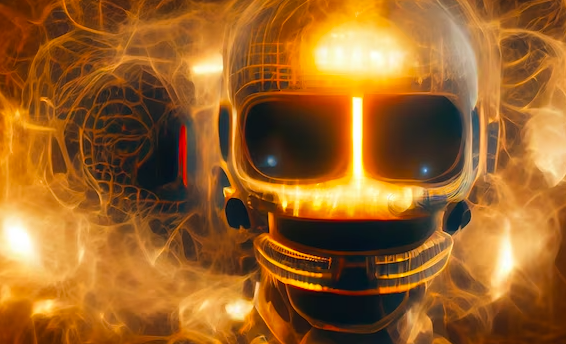
Artificial Intelligence (AI) has made remarkable strides in recent years, revolutionizing industries from healthcare to finance. However, beneath the surface of these impressive accomplishments lies a hidden danger: AI bias. As machine learning algorithms become increasingly integrated into our daily lives, it's crucial to understand the intricacies of AI bias, its consequences, and how we can mitigate it. In this article, we'll delve deep into the world of AI bias, uncovering its hidden dangers.
Table of Contents
- Introduction
- Understanding AI Bias
- Defining AI Bias
- Sources of Bias in AI
- Consequences of AI Bias
- Impact on Fairness
- Legal and Ethical Implications
- Unveiling Bias in AI Systems
- Real-world Examples
- Bias Detection Methods
- The Role of Data in Bias
- Data Collection
- Data Labeling
- Mitigating AI Bias
- Algorithmic Fairness
- Diverse and Inclusive Data
- The Responsibility of Developers
- Ethical AI Development
- Bias-Aware Algorithms
- AI Bias in Healthcare
- Diagnostic Bias
- Treatment Bias
- AI Bias in Criminal Justice
- Sentencing Bias
- Predictive Policing Bias
- AI Bias in Finance
- Credit Scoring Bias
- Investment Bias
- AI Bias in Hiring
- Resume Screening Bias
- Interviewer Bias
- Future of AI and Bias
- Regulatory Measures
- Research and Development
- Conclusion
- FAQs
- What is AI bias?
- How does AI bias affect individuals?
- Can AI bias be completely eliminated?
- Are there any regulations to address AI bias?
- What can individuals do to combat AI bias?
Introduction
Artificial Intelligence has permeated nearly every aspect of our lives, from recommending movies on streaming platforms to assisting doctors in diagnosing diseases. However, as AI becomes more ubiquitous, the issue of bias within these systems has gained prominence. In this article, we will explore AI bias comprehensively, understanding its origins, consequences, and how it affects different domains.
Understanding AI Bias
Defining AI Bias
AI bias refers to the presence of systematic and unfair discrimination in the outcomes produced by machine learning algorithms. It occurs when algorithms make decisions that favor or disfavor certain individuals or groups based on protected characteristics such as race, gender, or age.
Sources of Bias in AI
Bias in AI systems can originate from various sources, including biased data, biased algorithms, and biased human decisions during the development process.
Consequences of AI Bias
Impact on Fairness
AI bias can lead to unfair treatment of individuals, perpetuating stereotypes and discrimination. It can exacerbate existing inequalities in society.
Legal and Ethical Implications
The presence of AI bias raises significant legal and ethical questions. It may violate anti-discrimination laws and result in legal consequences for organizations deploying biased AI systems.
Unveiling Bias in AI Systems
Real-world Examples
Numerous instances of AI bias have been documented, such as biased facial recognition technology that misidentifies individuals of certain ethnicities more frequently.
Bias Detection Methods
Researchers are developing methods to detect and quantify bias in AI systems, aiding in the identification and mitigation of biased algorithms.
The Role of Data in Bias
Data Collection
Biased data used to train AI models can perpetuate bias. It's essential to collect diverse and representative data.
Data Labeling
Human bias can creep into the data labeling process, impacting the model's performance.
Mitigating AI Bias
Algorithmic Fairness
Developers are actively working on creating algorithms that are more fair and transparent, reducing bias in AI decision-making.
Diverse and Inclusive Data
Using diverse and inclusive datasets can help reduce AI bias, ensuring that the algorithms represent all demographics fairly.
The Responsibility of Developers
Ethical AI Development
Developers bear the responsibility of creating AI systems that prioritize ethical considerations and minimize bias.
Bias-Aware Algorithms
Developers should proactively incorporate bias detection and mitigation techniques into their algorithms.
AI Bias in Healthcare
Diagnostic Bias
In healthcare, AI bias can lead to misdiagnoses or inadequate treatment for certain patient groups.
Treatment Bias
AI systems may recommend treatments that are more favorable to specific demographics, leading to unequal healthcare outcomes.
AI Bias in Criminal Justice
Sentencing Bias
In criminal justice, AI algorithms can contribute to sentencing disparities based on race or other protected characteristics.
Predictive Policing Bias
Predictive policing algorithms can reinforce biased policing practices, leading to over-policing in certain communities.
AI Bias in Finance
Credit Scoring Bias
Biased credit scoring algorithms can limit financial opportunities for marginalized groups.
Investment Bias
AI bias in investment decisions can perpetuate wealth disparities.
AI Bias in Hiring
Resume Screening Bias
AI systems used in hiring can favor certain candidates over others, perpetuating discrimination in employment.
Interviewer Bias
Bias in AI-driven interviews can lead to unfair hiring decisions.
Future of AI and Bias
Regulatory Measures
Governments and regulatory bodies are considering measures to address AI bias, including transparency requirements and audits.
Research and Development
Ongoing research aims to develop better tools and techniques for identifying and mitigating AI bias.
Conclusion
AI bias is a pressing issue that must be addressed as AI systems continue to shape our lives. It's essential for developers, researchers, and policymakers to work together to create AI that is fair, transparent, and accountable. By understanding the complexities of AI bias and taking proactive measures to mitigate it, we can ensure that AI benefits all of humanity.
FAQs
What is AI bias?
AI bias refers to the presence of systematic and unfair discrimination in the outcomes produced by machine learning algorithms.
How does AI bias affect individuals?
AI bias can lead to unfair treatment, perpetuating stereotypes, and exacerbating inequalities.
Can AI bias be completely eliminated?
While it can be mitigated, completely eliminating AI bias is challenging due to the complex nature of the issue.
Are there any regulations to address AI bias?
Governments are considering regulatory measures, including transparency requirements, to address AI bias.
What can individuals do to combat AI bias?
Individuals can raise awareness, support unbiased AI development, and advocate for ethical AI practices.
In this article, we've explored the hidden dangers of AI bias, its origins, consequences, and the steps being taken to mitigate it. As AI continues to shape our world, it's our responsibility to ensure that it does so in a fair and unbiased manner.